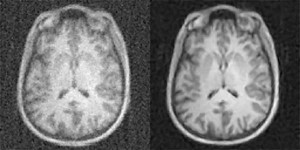
Medical imaging is used to detect everything from fractures to cancer. The computed tomography (CT) Scan Market in North America was worth more than USD 2.4 billion in 2019, with more than 80 million scans (CT scans) performed each year. According to a recent research report the medical imaging markets will grow 4% through 2027, with CT scans expected to grow at the fastest rate during this period.
Because medical imaging is fundamental to everyday healthcare, experts are concerned about the increase in the use of higher radiation–dose tests, such as CT and nuclear imaging because radiation exposure may lead to cancer later in life. The higher level of radiation, the higher level of image clarity, and the easier and quicker it is to read the scans. With today’s patient-focused clinical care, concerns about lowering radiation risk from routine tests such as mammography, general x-rays, dental x-rays, as well as higher radiation imaging CT scans are driving innovation in the industry.
The plan today in radiography is to use lower dose radiation to protect patients from being overexposed. Radiation exposure during an x-ray or CT scan depends on the targeted tissue or organ and the patient’s sensitivity to the radiation. The older you get, the less sensitive your body is to radiation, so it is essential to limit radiation exposure in children, teens, and young adults whose bodies are still growing.
The software behind the imaging equipment can also control radiation levels. Technicians can adjust the dosage depending on what they are imaging. However, there are challenges with lower dose images. Low-dose images look different; they are noisier (typically salt-and-pepper noise or mottle), have more visual distortion, and as a result, lower diagnostic certainty.
Salt-and-pepper noise (also called mottle or quantum noise) is prevalent in x-ray imaging. It is a random process due to fluctuations in the number of photons reaching the detector from point to point. Other sources of noise are also present, such as electronic noise, for example.
An accurate read of reduced-dose scans requires more time, training, and experience and is prone to diagnostic errors. Many hospitals outsource this task to specialists, which takes additional time to get a diagnosis. At any given time in the UK, more than 300,000 x-rays are waiting longer than 30 days for analysis.
Better image quality from low dose X-Rays
New technology innovation in deep learning is bringing more clarity to low dose medical imaging and diagnostics.
Deep learning can do far more than classification; it is also used for image processing. An image-to-image network is a special type of neural network that is trained to process input images and generate output images. When an image enters into the neural network, another image is created based on what the network has learned.
To train the neural network, you need a collection of high-dose (low-noise) images as the ground-truth dataset. This dataset typically contains hundreds of sample images that you want your neural network to recognize as “ideal” images. You will also need a data set of low-dose (high-noise) images so that the network can learn to recognize the noise distribution.
During training, the neural network will learn the type of noise, which is in the low dose x-ray, by comparing it with the higher dose, low-noise image.
Deep learning has the advantage of being able to learn various distributions of noise without having to engineer specialized filters, as used to be the case with traditional image processing. It is then possible to filter new noise distributions by adding more samples to the dataset and retraining the neural network.
In addition to noise reduction, deep learning can also be useful to increase the resolution of x-ray images via a super-resolution algorithm. In practice, a high-resolution x-ray is challenging to achieve due to the technological challenge and cost of making x-ray detectors with small pixel sizes and large fields-of-view. Super-resolution is the process of upscaling and or improving the details within an image. A low-resolution image is used as an input, and the same image is upscaled to a higher resolution, which is the output. The details in the high-resolution output are filled in where the details are virtually unknown. Applying super-resolution based on deep learning allows for increased overall image resolution and improved (or more accurate) diagnosis.
Finally, improving image contrast is another type of image enhancement achieved through deep learning. Poorly contrasted x-ray images are common in radiology as a consequence of various parameters of the imaging system.
Image-to-image neural networks are trained to perform all image enhancement operations at the same time, i.e., noise reduction, super-resolution, and contrast improvement. As long as the training image set is prepared correctly, a single neural network will be able to learn how to correct all these image imperfections at one time. Ideal (ground-truth) images need to be low-noise, high-resolution, and high-contrast.
Life-changing intelligence
Medical imaging is only useful when it contributes to a medical diagnosis. Image processing aided by deep learning will not replace medical professionals; it will assist them in providing a quick and accurate diagnosis.
Today, image-based diagnostics is a manual process and relies on the subjective analysis of the technician or doctor reading the image. Low-dose imaging requires specialists trained to read images and typically takes longer for a diagnosis. With deep learning, low-dose images are dramatically improved, reducing the need for specialized training. Patients receive a faster and more accurate diagnosis for better outcomes with less risk.
Written by Bruno Ménard, Eng., M.Eng. is a Software Director for Vision Solutions at Teledyne DALSA.