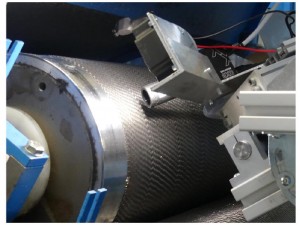
Although not widely used outside the textile industry, cloth carding machines are instrumental in cleaning, parallelizing, and blending raw fibers such as cotton and wool while eliminating impurities and short fibers. Carding produces a continuous web suitable for manufacturing using sharp wire teeth embedded in sturdy material covering fast-moving rollers or flats. Continuous exposure to abrasive raw fibers results in a loss of sharpness of the wire teeth. In turn, this affects the fiber transport process during carding and reduces the quality of the nonwoven fabric to the point where it is unusable. Cleaning and replacing cards are expensive in labor, parts, production downtime, and wasted raw materials.
Industry 4.0 has come to the rescue of the textile industry, bringing predictive maintenance (PdM). Leveraging Artificial Intelligence (AI) and Machine Learning, PdM anticipates when specific parts will fail so replacement can be proactively timed so as not to delay production.
Recently, the Faserinstitut Bremen Research Institute (Bremen, Germany) and the Chemnitz University of Technology (Chemnitz, Germany) teamed up to develop an Industry 4.0 PdM digital monitoring system for the textiles industry. It aims at observing degrees of wear of card clothing over time and offers new possibilities for maintenance prediction and textile production planning.
The project harnessed the power of machine vision and AI to analyze wear in card tooth geometry, tip shape, and density, all of which are factors in carding efficiency. Central to the imaging system was an SVS-Vistek exo541MGE 20.3 megapixel CMOS GigE Vision camera, equipped with an Opto Engineering MC3-03X lens, and mounted in an IP67 housing to prevent damage by carbon fiber dust. Power Over Ethernet (PoE) single cable delivery of power, data, and controls simplified deployment for testing. The image area observed was approximately 25 x 25 mm² at 4504 x 4504 pixel resolution, corresponding to an edge length of 5.5 μm per pixel. This setting enabled observation at a nearly microscopic scale. The camera's position was 2 mm above the tooth tips, a sufficient distance to prevent damage to the camera or the clothing during testing.
Mimicking real-world environments, the camera was mounted on a rack constructed from aluminum profiles that allowed it to be positioned over production lines featuring widths up to 3 meters. A stepper motor enabled camera focusing by remote control. An illumination unit with 800-lumen luminous flux was directly attached to the camera holder. System software was self-developed based on the OpenCV SDK applying its findContours function.
The system was tested in a pilot plant line at Sächsisches Textil Forschungs Institut in Chemnitz (STFI) and later on the lines of three partner companies. According to the researchers, by the end of the project, the imaging system reached a state of "proof of concept". The only drawback was that partner companies found that the camera needed to be positioned over an existing line while maintenance doors were opened. Regardless, since the doors needed to be opened during cleaning or maintenance intervals, this schedule was sufficient to observe any increase in wear.
Achieving a higher level of AI performance will require further development. This includes building an extensive database for cylinder geometries and card clothing types, data about typical wear patterns, and the limits of clothing usability. Once completed, this database will be combined with data for optimal camera positioning for each occurring combination of cylinder geometry and clothing used on it.