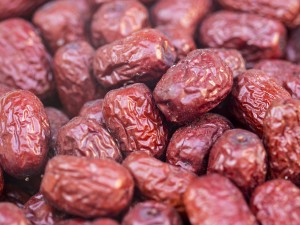
How long do you inspect an apple at the store before it ends up in your basket? Today’s consumers expect their food products to be high quality on a consistent basis. That’s why companies such as Ocean Optics and its customers are using advanced optical sensing tools and analytics to improve the quality of food for consumers, and the quality of solutions for food processors and sorting machine manufacturers.
Historically, food sorting has been managed manually, relying on the expertise of workers to judge quality visually. The introduction of machine vision systems, optical scanning and spectroscopy has added a much deeper level of understanding and analysis. For example, some spectrometers can “see” inside a fruit peel to determine fat, protein or water content, or to spot internal browning, rot or bruising.
New tools such as machine learning add another layer of analytical capability. For example, by fusing spectroscopy with advanced statistical models and machine learning architecture, different groups within the supply chain can realize immediate benefits: for sorting machine integrators, analytical options are now more precise and reliable; for food processors, more efficient facilities are achievable; and for consumers seeking quality produce, smarter, more accurate sorting and grading inspire confidence in what shows up in their basket.
Date fruit sorting case study
(Figure 1 shown above).
Lugo was one of the first companies to develop sorting technology for dates, in 2003, and continues to develop innovative technologies for identifying internal quality aDates have been a popular fruit since biblical times. Consumers know exactly the kind of date they want: not too wet and not too dry.
Ocean Optics was approached by Lugo Machinery & Innovation, a leading supplier of fresh produce sorting products based in Israel, to improve their manual method of sorting dates by moisture nd defects of fruit.
Lugo’s goals for the date sorting project were simple: first, to automate the sorting process to eliminate all manual inspection; second, to perform the measurements rapidly; and last, to make the measurements non-destructively. Lugo’s timeline was very short, with only four months from project inception until date harvest season; their limited knowledge of spectroscopy was another challenge.
Feasibility tests were performed on date samples from Lugo, which quickly showed NIR correlations to moisture levels in the fruit, helping to determine the choice of system hardware. This setup was used at the customer site to analyze a much larger sample set, then used for training data to develop proprietary machine learning algorithms.
Lugo anticipated date moisture response between 850-900 nm and imagined the analysis would focus solely in that region. But based on Ocean Optics’ experience analyzing other fruits and vegetables, we extended the analytical range to include broader patterned spectral features, which would help us to develop machine learning algorithms. This approach -- broadband versus discrete wavelength spectral analysis – yields more accurate results and makes the data less susceptible to deviations related to optical interference.
Diving deeper into data with machine learning
Applied analytics also factored into the Lugo date sorting project. Having shown the robustness of algorithms developed earlier, as well as their predictive accuracy, Lugo integrated the spectral platform into a conveyor-belt system with the algorithms running on a devoted PC. The architecture of this sorting system scans each date and weighs over 12 potential correlation models, ultimately “voting” on the best model and generating the output in milliseconds. Classification methods were valuable in this application, which required broader threshold decisions versus precise numerical outputs; some of these methods include k-nearest neighbors (k-NN), Gaussian, and polynomial. The method toolbox is evolving to include regression models for accurate quantified outputs, which is invaluable to those working in process streams looking for impurities from any number of sources.
By drawing mathematical correlations between absorbance and concentration trends we have taken the first step toward advanced understanding. As these correlations evolve from linear fits to more complex functions, understanding is further advanced, and as these complex functions begin to account for multiple species, we advance further still. But at some point, a wall is hit; at some point there are so many interconnected inputs working to generate so many outputs that traditional deconvolution methods become daunting if not impossible.
Introducing machine learning to the fruit sorting process brings a deeper level of analysis that is not relying on the human eye and perception but is rather folding its fingers through every digital bit of data, statistically analyzing the entire data array of each spectrometer scan at every pixel on the detector. This system can now see abnormalities previously missed and develop smarter, more streamlined correlations for far more intertwined variables.
One of the most powerful aspects of machine learning is the ability of non-experts to introduce new training sets to the system and achieve results previously attainable only to experts in the field. This creates the freedom to develop beyond the initial product capabilities without the cost of an expert for such expansions and evolutions. With these new tools, what was once highly intimidating and restricted is now entirely approachable to users with different levels of expertise (Figure 2).
Figure 2. Robust spectral tools and statistical modeling can help predict flaws affecting fruit quality, damaging brand reputation and resulting in lost business.
Various communication interfaces are available to work with PLCs and common process equipment. In our case study, Lugo and Ocean Optics engineered the spectrometer module to communicate with the system PLC for triggering action to a valve that steered the dates in the correct direction (appropriate moisture category). This functioning system created a beta platform to refine and optimize the algorithms before final implementation.
Applied insight at work
Today, the fully integrated Lugo system scans five dates per second and is entirely automated. This has reduced overhead costs, improved safety and allowed Lugo to focus on refining other aspects of the sorting process. Also, with advanced statistical models now established, the customer will be able to develop additional analyses without the need for modeling expertise.
With the fusion of spectroscopy, statistical modelling and machine learning architecture, sorting machine integrators and food processors can build more efficient sorting and grading systems.
Written by Derek Guenther, Senior Application Scientist, Ocean Optics