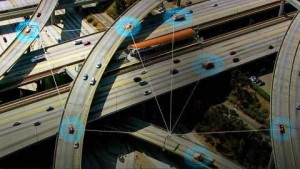
One of the important questions surrounding the deployment of new technologies in autonomous vehicles is which type of sensor, or sensor combination, will provide the optimum price and performance. The issue is complex because the sensors chosen to perform specific tasks can be stipulated only after the capabilities of the entire systems used to control the vehicle have been characterized.
An autonomous vehicle can be defined as comprising three major system components. The first is responsible for sensing the environment surrounding the vehicle. The second maps the environment surrounding the vehicle, which then enables it to be able to determine its location at any given time. The third is accountable for the decision-making capabilities of the autonomous vehicle under various driving scenarios.
From the current research undertaken at the major automotive companies and numerous third-party suppliers, there appears to be a consensus that multiple sensor types will need to be deployed in an autonomous vehicle to sense and map the environment. Engineers at Robert Bosch, Aptiv and Continental are all of the opinion that autonomous vehicles will need to capture data from radars, cameras as well as Light Detection And Ranging (lidar) systems. These sensor technologies complement each other and provide the best possible reliability in day and night, and in rain or fog. Indeed, developers at major automotive companies, such as Ford, General Motors, Volkswagen and Nissan would appear to concur, since they have all demonstrated autonomous vehicles that employ all of the sensor types (Figure 1).
Figure 1: Both GM (top) and Ford (bottom) experimental autonomous vehicles have used multiple sensors including cameras, radar and lidar.
Cameras, lidar and radar
Typically, cameras comprise the heart of most automotive sensing systems, enabling images in the full 360 degree field-of-view (FOV) of the vehicle’s surroundings to be captured. Nevertheless, short-range radar (SRR) working in the 24GHz band can also be employed, as can long-range radar (LRR) systems in the 77GHz band. Lidar systems on the other hand, can be used to deliver real-time 3D data with up to 0.1-degree vertical and horizontal resolution with up to a 300-meter range and 360 degree surround view.
When employed in a vehicle, lidar systems can capture a dense point cloud of 3D data from both fixed and moving objects. Radar systems, which emit and receive radio waves rather than light, are complementary to lidar because they can also be used to provide data on the velocity and bearing of objects which have low light reflectivity. Long-range radar sensors can track high-speed objects, such as oncoming vehicles, while short-range radar sensors provide detail about moving objects nearer the vehicle. Cameras, on the other hand, can measure the light intensity reflected off or emitted from objects, adding to the detail of the objects themselves.
Building an autonomous vehicle that incorporates all the aforementioned sensor types ensures that the inherent technical limitations of one sensor type are countered by the strengths of one or more of the others. If such an approach is taken, however, the issue then becomes one of what sort of on board processing model must be developed to process the large volume of data captured by the sensors.
Fusing data
One approach to the conundrum is to fuse together the data from the multiple sensor sources on the vehicle together, spatially, geometrically and temporally aligning the data prior to processing which results in a large single sensing system. The approach would enable an on board processor or processors to estimate the state of an autonomous vehicle when observations from the individual sensors fail to be accurate enough. An alternative is to engineer multiple independent sensor processing systems, each of which can support fully autonomous driving on its own. Whatever approach is taken, however, a versatile, redundant, and fail-operational systems architecture is needed to make automated driving a reality.
For their part, engineers at Bosch are developing a network for automated vehicles that combines data sourced from all the sensors in the vehicle in a process called sensor fusion. The sensor data is assessed by an electronic control unit in the vehicle to plan the trajectory of the vehicle. To achieve maximum safety and reliability, the necessary computing operations are done by a number of processors working in parallel.
Engineers at Intel/Mobileye, however, are taking a somewhat different approach in an effort to overcome what they perceive as non-scaleable, and therefore, expensive aspects of an autonomous control system (Figure 2).
Figure 2: Intel/Mobileye have proposed a formal, mathematical model to help ensure that an autonomous vehicle is operated in a safe manner. The Responsibility-Sensitive Safety (RSS) model provides specific and measurable parameters for the human concepts of responsibility and caution and defines a "Safe State" designed to prevent a vehicle from being the cause of an accident, no matter what action is taken by other vehicles.
Multiple sensing systems
They have already demonstrated an autonomous vehicle fitted out with only cameras, as part of a strategy that will involve the creation of what the company calls a “true redundant” system. Now, they intend to build a sensing system consisting of multiple independently-engineered sensing systems, complementing the camera-based system with one also built using radar and lidar. While the fusion of the data from the cameras will be used to localize the vehicle, the fusion of the radar and lidar data will be used at the later stage of planning the trajectory of the vehicle. Each of the systems will be able to support fully autonomous driving on its own, in contrast to initially fusing raw sensor data from the camera and radar/lidar sources.
Aside from sensing the environment surrounding the vehicle, the suite of sensors fitted to autonomous vehicle will also be responsible for mapping the environment, enabling a vehicle to determine its location at any specific time. One approach that has been taken to do so is to record a cloud of 3D points previously obtained by a lidar to create a map and then to localize the position of the vehicle by comparing the 3D points obtained by the lidar in the vehicle with those on the map. An alternative approach, and one espoused by Intel/Mobileye, is to make use of the large number of vehicles that are already equipped with cameras and with software that can detect meaningful objects surrounding the vehicle. Such an approach would result in the creation of a map based on crowd sourcing that could then be uploaded to the cloud. Autonomous vehicles would then receive the mapping data over existing communication platforms such as a cellular network.
With such a large amount of data captured by numerous sensors, a new vehicle computer infrastructure may be required to enable the volume of data to be transferred within the vehicle. According to James Hodgson, a Senior Analyst Autonomous Driving at ABI Research, the volume of data generated by multiple types of sensors such as cameras, radars, lidars as well as ultrasound systems can reach 32TB every eight hours. To define an infrastructure that can handle such high data rates, Aquantia, Bosch, Continental, NVIDIA, and Volkswagen have established the Networking for Autonomous Vehicles (NAV) Alliance. Working together, the companies plan to bring multi-gigabit/s Ethernet networks inside an autonomous car whilst addressing the challenges related to noise emission and immunity, power consumption, reliability, and safety standards.
Data processing by on-board system
Lastly, of course; and validating and certifying for safety., there is the issue of how the on-board system in the vehicle computer architecture processes the data from the sensors in order to enable the vehicle to maneuver appropriately under various driving scenarios. It is not enough for the vehicle to be able to sense its environment and be able to localize its position within it, it must also be able to plan and act on the data acquired.
Needless to say, it is here that the role of artificial intelligence (AI) systems are becoming increasingly prevalent, enabling vehicles to acquire complete knowledge of the numerous potential traffic conditions that may occur. Such systems learn from traffic situations in a process known as ‘deep learning’, and draw their own deductions from which they can deliver a course of action. However, because such AI systems operate probabilistically, there are concerns that their reliability may be insufficient.
For that reason, companies like Mobileye are adding a separate, deterministic software layer on top of their AI-based decision-making solution. Mobileye’s Responsibility Sensitive Safety (RSS) model formalizes human notions of safe driving into a verifiable model with logically provable rules, defines appropriate responses, and ensures that only safe decisions are made by the automated vehicle. The model itself is responsible for validating the trajectory of the vehicle based on the results from a trajectory planning system which creates a plan of action based on data acquired by the sensors on the vehicle.
Validated and certified for safety
Clearly though, whatever software is employed in autonomous vehicles, it will need to be validated and certified to ensure that the decisions made by the autonomous vehicle are safe. Indeed, due to the numerous potential driving situations that an autonomous vehicle is likely to encounter, the challenges involved in verifying that the software used in such vehicles can be used with an appropriate level of safety may be one of the greatest challenges that engineers face. Especially considering that many of the AI software systems used are non-deterministic in nature and therefore difficult to test.
In Europe, however, two such projects to do just that are underway. One such effort has been launched at The German Research Center for Artificial Intelligence (DFKI) and TÜV SÜD. Engineers there are developing an open platform, known as ‘Genesis’, for OEMs, suppliers and technology companies to validate artificial intelligence modules and thus provide the basis for certification. Another project, this time in the UK, has been funded by UK Research and Innovation. Dubbed Smart ADAS Verification and Validation Methodology (SAVVY), the project partners intend to develop techniques to enable the robust design and verification and validation of autonomous software features in a safe, repeatable, controlled and scientifically rigorous environment.
So while some vendors may tout that the technical challenges of creating a truly self driving vehicle has been solved, it is still the case that self-driving functionality is still dependent upon extensive software validation, not to mention regulatory approval. And until those issues are also overcome, the fully autonomous vehicle itself may still be a few years away.
Written by Dave Wilson, Senior Editor, Novus Light Technologies Today