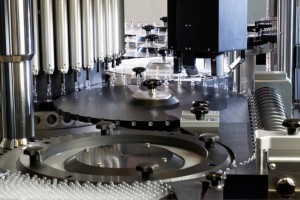
Inspecting containers of medicinal products for defects is vitally important to ensure that they meet the demanding quality requirements of a manufacturer prior to shipment. While such inspection was originally carried out manually, today highly automated vision systems perform the quality assurance function many orders of magnitude faster and with a higher degree of accuracy than a human operator.
Over the past few years, many such automated inspection machines have been developed at Seidenader, a vision integration specialist based in Markt Schwaben in Germany.
Typically, these machines use one or more cameras to capture images of medical vials or containers, and process the images using classical software-based image analysis techniques to determine whether or not any cosmetic defects or unwanted particles are present. Any defective containers that are found by the systems are then rejected from the production line.
Despite the effectiveness of such traditional vision-based software techniques, developers of vision-based inspection machines such as Seidenader are continually investigating methods to improve the performance of their image recognition algorithms. That is to ensure that their systems are as effective as possible at performing the real-time quality control function in the production environment.
Machine learning
To determine whether a software identification system based on machine learning techniques might be more effective at inspecting the quality of the vials than more traditional classical approaches, Seidenader collaborated with Germany-based consultancy d-fine and chartered them with the goal of evaluating a number of machine learning architectures and algorithms that could potentially prove useful once deployed on machine vision systems in the quality control environment.
As a reference from which to judge the effectiveness of identifying defective containers using a new deep-learning software approach, a classical machine learning (ML) software architecture was first developed by the d-fine engineering team. The results from the new deep-learning approach were then compared with the traditional ML technique to judge the relative effectiveness.
According to Dr. Tassilo Christ, a Senior Manager at d-fine, classical machine learning software is a two-stage process. In the first stage, features of the images in question are encoded, whilst in the second learning stage, the software learns to discriminate between different object categories.
Photographic, or scanned images, constitute a high dimensional representation of the categories (or object classes) to be recognized. A large variety of images will also be captured during the inspection process. In the classical ML approach, the d-fine team reduced the dimensionality of the images to a lower order representation that still captured the essence of the information contained within them using a Histogram of Oriented Gradients (HOG) transformation. This well known algorithm describes the local object appearance and shape within an image by the distribution of intensity gradients or edge directions.
Once the lower dimensional features representing each image were encoded, they could then be presented to a support vector machine algorithm. Given a set of training examples, each marked as belonging to one or the other of two categories – either an acceptable vial or a reject – the SVM training algorithm then built a model that assigned new examples presented to it into one category or the other.
Deep learning
By contrast, the deep learning system applied by the d-fine team is only a one stage process. Unlike the more classical machine learning approach, with the deep learning system it is no longer necessary to extract the features from the images, because when parameterized properly, the software system will perform what is called one-step “end to end learning” (Figure 2).
Figure 2: Two machine learning approaches were evaluated by the d-fine team -- an SVM system and one based on Convolutional Neural Networks.
According to Dr. Christ, the secret to doing so is to provide an appropriate initialization and intelligent training algorithm and a proper cost function by which to penalize the deep learning network if it should incorrectly categorize an image. Having done so, the system itself can extract those features of the images by which it can distinguish a good product from a bad one without a dedicated feature-engineering step.
The deep learning system employed by the d-fine team comprises a convolutional neural network (CNN) that is highly effective at end to end learning especially for image recognition tasks. CNNs are best imagined as a series of layers each which perform mathematical transformations to the input data and during training adapt themselves to the image characteristics.
These transformations comprise the convolution of the inputs with a kernel function that is being adapted during the training phase, computation of a nonlinear activation function and spatial pooling of neural activations.
Thereby, deep learning system learns to represent the features in the input data in a hierarchical fashion - with simple, generic features being represented by layers close to the input and higher more specific abstractions corresponding to the activations in the deeper layers of the network.
Learning this hierarchical representation requires a lot of data and computing power due to the need to adapt the internal parameters of the network (mainly the filter functions) in an iterative process – a feature shared by all deep learning approaches. After the training has finished, the computational requirements are much less demanding, because the classification is achieved by a simple “forward-pass” of the image through the network, where the trained classifier assigns a probability for what the object in the image is.
To summarize, the CNN can not only identify similar objects in an image but, by finding the right features in the image autonomously, effectively learns by itself what makes the objects shown similar or different.
Training and testing
To determine the effectiveness of the two approaches, a fixed set of pre-classified images of filled drug containers was used to train and test both a classical approach based on a Support Vector Machine and the deep learning CNN setup.
The decision taken by the classifiers for a given vial image falls into one of four categories: True Positives (TP) represent vials that where labelled as rejected by the human operator and also correctly so by the classifier. False Positives (FP) are labelled “accepted” by the operator but are falsely rejected by the network. False Negatives (FN) and True Negatives (TN) are defined in analogous manner. Using these quantities it was then possible to determine the accuracy, precision and recall of the two approaches as shown in Figure 3.
Figure 3: To fully evaluate the effectiveness of any model, it is important to examine accuracy, recall and precision. While the accuracy of a system might be self-explanatory, the precision of the system measures the purity of the image sample rejected by the system. Recall indicates the detection efficiency of vials with insufficient quality.While the Accuracy of a system might be self-explanatory, the Precision of the system provides a measure of the “purity” of the rejected samples, while the Recall of the system corresponds to the “efficiency” of the removal of vials with insufficient quality.
The accuracy of the HOG/SVM combination was found to be just 95% with a precision of 90.91% and a recall of 100%. With a de-noising algorithm applied to the images, the performance improved. The accuracy of the system rose to 98.33%, the precision to 96.77% whilst the recall remained unchanged at 100%. However, neither approaches compared in performance to the deep neural network algorithm. Here, the network achieved almost perfect classification after some data augmentation. While the accuracy of the system was 99.83%, the precision of the system was 99.67% and the recall 100%.
Having proved the effectiveness of the deep learning algorithm, the software was rolled out on a virtual inspection machine where it proved possible to classify vials of drugs in real time. The results are now being analyzed by the production team at Seidenader, who are comparing the effectiveness of the software with their more traditional approaches with an aim to possibly deploying the software in a real production environment in the future.
While Dr. Christ believes that such machine learning techniques can prove invaluable in vision-based inspection system, he strongly advises those unfamiliar with the subject to seek professional advice before embarking on employing such software in a system.
While machine learning can be of benefit, he warns that such software systems can lead to miserable failure if they are applied without a proper understanding of the nature of the specific image data that needs to be analyzed and without careful consideration of the appropriate software to solve the problem at hand.
Written by Dave Wilson, Senior Editor, Novus Light Technologies Today